Meta-Analysis for Comparing Apples and Oranges
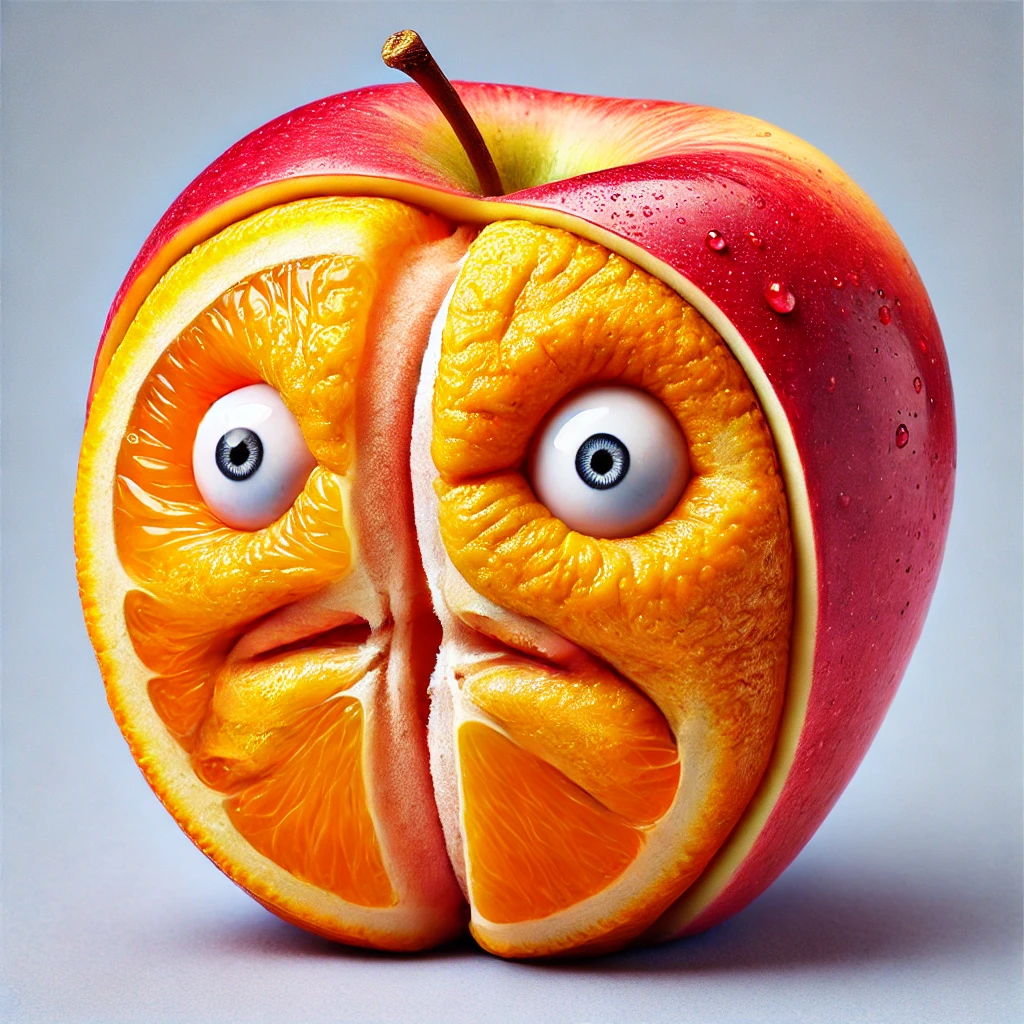
This is how I kept myself busy waiting for conda packages to install last month :( .1
Every decade, we find a new reason to doubt the truth-value of what we learned in grad school comparative politics and IR seminars. In the 2000s or early 2010s, it was the credibility (or causal identification) revolution in social science. A decade before that, the problem was contextual: The end of the Cold War, the Unipolar Moment, and the third wave of democratization upended a lot of what political scientists “knew” about the world.
Today, two major concerns dog quantitative research in IR and comparative politics: 1) Insufficient power, and 2) external validity.
Recent work in the Journal of Politics and American Political Science Review raise concerns about the statistical power of most political science research. Near universal under-power interacts with the file drawer problem—non-significant findings don’t get published—to virtually guarantee that published findings over-estimate the true magnitude of the effects they claim to find.2 Power concerns started as the preoccupation of experimental or survey researchers, but they are no less real in observational research. Of course, the standard remedies are different. If, for example, you are estimating the effect of democracy on economic growth—see the above-mentioned APSR article by Doucette—you can’t simply enroll more units in your study to increase power. Some people argue that this makes certain areas of research “Fundamentally Unanswerable Questions” or FUQs.3 Others counter that ~important~ questions still ought to be answered, even if they are not amenable to high-powered designs. My personal view falls somewhere in between, but I’m going to move on to problem 2.
The second issue is external validity. As Tom Pepinsky has noted, modern comparative politics research often prioritizes internal validity and design-based inference in single-country studies: This trend is visible in IR too, especially with survey experiments. What separates this type of research from “area studies,” which political scientists are not supposed to do?4 The authors (including me) hint at or make claims about generalizability to other contexts, but usually do not test those claims. As with power, the discussion of external in-validity is particularly vibrant in the experimental literature. Experiments provide leverage to estimate the causal effect of some treatment without bias within a particular sample, but as Deaton and Cartwright and many others point out, randomization itself does not provide any weight to claims that extend beyond the boundaries of a particular trial.
Practically speaking, there is often a tradeoff between internal and external validity. Internally-valid experiments (natural or artificial) are possible in settings that are atypical, and nothing in the standard design based inference toolkit (i.e. Mostly Harmless Econometrics) gives us leverage to determine the extent to which the result depends on those atypical features. Some scholars—like Naoki Egami, Erin Hartman, Tara Slough, Scott Tyson, and others—have recently advocated for a “bounds” based approach to assessing generalizability, but they do not offer an estimator for the external validity of a single-site study. A useful estimator of this type might not exist.
The Meta Analysis Fix?
People have started to suggest that meta-analysis will be part of the solution to both problems: power and external validity. Meta-analysis aggregates results across multiple individual studies that aim to investigate a relationship between the same treatment
In comparative politics, the idea of using meta analyses for external validity is strongly associated with the MetaKeta projects coordinated by Evidence in Governance and Politics (EGAP). The MetaKetas coordinate multiple field experiment teams to deploy harmonized interventions and outcome measures in around a half dozen sites (in different countries in the Global South), and then conduct a meta-analysis of the results to draw generalizable conclusions about the effects of interventions like accountability information provided to voters, or the introduction of community policing.
The idea that meta-analysis can address power problems is maybe newer (see Alex Coppock’s recent thoughts on BlueSky). Here, the idea is to treat individual studies as potential replications of each other, not to understand the generalizability of effects across domains. If a low-powered study over-estimates the magnitude of some
This all sounds pretty good, if onerous.5 But the promises of meta-analysis only hold under certain assumptions. A nice article in the AJPS by Tara Slough and Scott Tyson (henceforth, S&T) lays out the meta-analysis assumptions in a way that makes it clear how hard they are to satisfy. Their argument, which is about external validity but applies equally well to power, boils down to this:
For meta-analysis to yield an estimate of a general causal effect, the included studies need to be target equivalent or aiming at the same estimand.6 This requires:
- Harmonized measures of the outcome
- Harmonized “contrasts” between treatment and control
- An assumption that the effect generalizes across settings
These are all very weighty assumptions. I will take them out of order for rhetorical effect. Third, and specific to external validity concerns, S&T demonstrate that meta-analyses assume the external validity of a mechanism, not test for it.7 To estimate a general causal effect via meta-analysis, the user needs to make a substantive, theory-driven assumption that the causal mechanism in question operates in all the settings of all included studies (S&T say this “ultimately relies on a subjective argument”).8
Second, meta-analysis breaks if the included studies do not use harmonized (i.e. conceptually identical) measurement strategies. A meta estimate combining results from a study that examines the effect of CBT on participation in crime and violence and a study that examines the effect of CBT on self-reported wellbeing would not be a valid estimate of any particular general causal effect. Seems correct.
First (third), and potentially the stickiest for applied comparative politics and international relations researchers, S&T show that target equivalence demands not only that the treatments are similar, but that the contrasts between treatment conditions are harmonized across studies. If treatment/control contrasts are not harmonized, then it’s not possible to say for certain that different studies produce different results due to statistical issues, as opposed to genuine differences in treatment effect across cases.
It is very difficult to harmonize treatments in studies about social, political, and behavioral processes. Take the meta-analysis by Bauer et al. which studies the relationship between exposure to war violence and pro-social (cooperative) behaviors. The authors take some care not to compare apples and oranges, showing in Table 1 that they exclude otherwise-eligible studies from the meta-analysis if they focus on other types of violence like criminal violence or election riots. The same table, though, shows that paper’s estimate of the general causal effect
Table 1 from Bauer et al. (2016)
Treatment similarity is only half of the harmonization assumption proposed by S&T. The full assumption is that the contrast between treatment and control conditions is harmonized across studies. This is an even higher bar for a lot of settings in which political scientists want to use meta-analysis. Treatment
The challenge is clear if you zoom in on a specific example, like the Community Policing MetaKeta. In this study, which deploys and evaluates community policing interventions in six sites in the global south, the harmonized contrasts assumption stipulates that the difference between “business as usual” and a community policing treatment in the largest province in Pakistan should be the same as the difference between “business as usual” and a community policing treatment in the Brazilian state of Santa Catarina, and so too for the Philippines, Uganda, Medellín, Colombia, and Monrovia, Liberia.
If the treatments are harmonized (which EGAP takes great pains to ensure), then this condition is only fulfilled if the “business as usual” experience of policing in the various sites is very similar. Looking just at Pakistan and Brazil for example, I would hazard that the control conditions have some pretty important differences. Those differences may even be large enough that the harmonized treatment (community policing) ends up de-harmonizing itself insofar as it has irreducibly different social meaning in the two contexts. The police force in the Brazil study, for example, is a heavily-armed military police organization operating in a context where the majority of World Values Survey respondents report “A great deal” (11.4%) or “Quite a lot” (42.7%) of confidence in the police. In Pakistan, the Punjab State Police are less militarized, probably less capable, and seen as less competent (“A great deal” (15.5%) or “Quite a lot” (27.0%)).
Policing in Brazil and Pakistan differs substantially—so does public trust in police—potentially altering the meaning of an intervention that increases police-society contact via increased beat patrols, community meetings, etc. It seems plausible that the effect of more police presence and police attempts to engage civilians might have different (even opposite) effects depending on baseline levels of trust and expectations of competence.
Meta-Analysis Fails Silently
What happens if you try to compare two studies that are not contrast harmonized? It will still be completely possible to produce a meta-analytic estimate of something, but it will be hard to say what exactly that estimate means.
Meta analysis relies on assumptions, and it is difficult (or impossible) to affirmatively verify that the assumptions are satisfied.10 The problem is, because these are conceptual issues not statistical issues, none of the techniques for doing meta analysis will “break” or warn you that you are comparing incommensurable studies. Ask the computer to compute the average of an apple and an orange, and it will. The result may not be useful, and it may be creepy (see above).
Three and a Half Incomplete Paths Forward
Slough and Tyson’s paper has convinced me that solving external validity and power issues isn’t as simple as throwing studies into the meta-analysis blender and hitting “pulse.” If anything, they understate how hard it is to identify harmonized treatment/control contrasts outside lab- and survey-experimental settings. So, what do we do in applied research? I don’t really know. But here are three and a half ideas—most of which address external validity more than power. On power, I’m essentially waiting for my FSU colleague Carlisle Rainey to figure it out.
1. Re-run
One option is brute force: Do the studies again, but bigger and in more contexts. Low-powered studies of attitudes and behavior could be re-run with more units. Issues with treatment contrasts or external validity could be mitigated by eliminating pure control conditions in experiments and scaling up multi-site trials.
This could work—to an extent. But who would do it? Large-scale replications are expensive, and political science career incentives typically don’t support specializing in checking other people’s work (so to speak). More fundamentally, re-running studies doesn’t solve the power problem for FUQs, where the world simply doesn’t provide enough units.
1.5. Re-interpret
Why do political scientists conduct underpowered studies in the first place? And why do even MetaKeta initiatives, designed to assess external validity, max out at half a dozen trials? Because conducting large studies is expensive and time-consuming, with diminishing returns. Absent a technological solution to the problem of difficulty, we might do well to change how we interpret results of studies that don’t have massive power or include all the contexts.
A study powered to detect a one-standard-deviation effect of some independent variable is useless for testing whether any effect exists. But if the treatment is costly, complex, or hard to scale, one might not care about small effects. A null result in a low-powered study certainly is not proof that no effect exists, but it is evidence that any true effect is smaller than the minimum detectable effect size—which may be the actual policy-relevant threshold.
We could reframe how we talk about external validity in a similar way. Many meta-analyses don’t estimate a true general causal effect because they aggregate studies with non-harmonized contrasts and shaky assumptions about external validity. But they do tell us something useful. The MetaKeta community policing study, for instance, doesn’t provide a universal causal estimate of the effect of community policing on trust. But it does tell us that an INGO implementing an off-the-shelf community policing program across highly diverse contexts in the Global South shouldn’t expect much movement in the legitimacy and performance indicators they might care about improving. That’s valuable information.8
Reframing research in this way might seem technical and arcane—but so did the language of causal identification at first. And yet, after decades of repetition, even normies now know that “correlation does not equal causation.”11
2. Retreat
Maybe we should stop expecting political and social relationships to generalize much at all. Maybe we should just study politics where we study them. In other words, maybe comparativists and IR scholars should own up to doing area studies. Investing in areas studies hasn’t hurt Americanists!
This approach has benefits. It reduces the incentives for conceptual stretching and (I think) aligns journal article claims more closely with researchers’ actual beliefs about generalizability. It also acknowledges that single-context findings can still be valuable.12
That said, I don’t think we should abandon generalizability altogether. Some major findings from the first century of political science clearly hold across time and space. For example, while democracy is a messy and abused concept, most political scientists agree that democratic and non-democratic systems function differently in meaningful ways. Abandoning generalizability altogether would be defeatist—but maybe we don’t always need to make universal claims.
For power problems, retreating would mean accepting that some studies simply can’t rule out the existence smaller causal effects. Sometimes that is fine! If the goal of social science is to generate knowledge that improves human well-being, then identifying large, impactful effects is more important than chasing minuscule ones.
Some development economists already approach this issue through cost-effectiveness analysis—evaluating whether an intervention’s impact justifies its opportunity cost. In this frame of mind, it’s not enough to ask whether intervention
Still, I have mixed feelings about retreating to address power concerns. Prioritizing large effects makes sense for applied research, but well-powered studies that identify smaller effects often contribute to theoretical progress. After all, the blockbuster weight-loss drugs (semaglutides) that are now transforming obesity treatment stemmed from 1980s research on gila monster venom. Abandoning basic science would be a mistake.
3. Re-focus
Most political scientists won’t respond to these “crises” of low power and low generalizability by shifting their careers toward replication studies or meta-science. So how do researchers proposing new hypotheses avoid the worst pitfalls of low-powered and over-generalized research?
My (deeply un-satisfying) answer: let theory guide research programs more explicitly. If we agree as a field (HAH!) that we really care about uncovering social truths that generalize across time and space, where should we start? We actually have theoretical reasons to expect that some political processes generalize better than others.
For example, certain cognitive and social processes—like fear and disgust, and like in-group affinity—appear to be pretty universal across cultures. I’m sure there are similar theoretically-universal things to be studied in political institutions as well. Because we have a theoretical basis for expecting these mechanisms to hold across settings, we can be more confident in the generalizability of findings related to them. To the extent that generalizability is our goal, we should focus on the types of mechanisms where we have a solid reason to be optimistic about generalizability.
This brings us full circle to Slough & Tyson’s critique of meta-analysis: If we want to use statistical aggregation tools to get ourselves out of trouble, we should focus our efforts on environments where their assumptions are plausible. That’s easier said than done, but what isn’t!
Before you ask, I was working on a system that wouldn’t let me install mamba………. ↩︎
The intuition here: low-powered designs have very large minimum detectable effects. If the true effect of some intervention is a 2 percentage point change in some outcome and your study is powered to detect effects as small as 20 percentage points, you will most likely find nothing and you will bin the study or an editor will bin it for you. However, if you observe an extreme result from the tails of the sampling distribution and find a statistically significant effect, you will put out an article estimating that the effect size (≥20pp) is at least 1000% larger than it really is (2pp). ↩︎
If you try to answer a FUQ, the enterprise is FUQed. ↩︎
In IR it’s totally ok to do area studies as long as the area is the United States ;). ↩︎
In a brief BlueSky exchange, Alex Coppock reminded me that hard work is part of the job. Point taken. ↩︎
They have a formal definition in the paper, of course. A pair of studies are target equivalent iff, given the same treatment contrast (
), the same measurement strategy ( ), and the same setting ( ), the treatment effect functions are equivalent but for statistical noise: ↩︎I think this actually follows pretty logically from the equivalence in the previous footnote, plus the observation that all estimates of
are rendered with some sort of sampling variability or noise. Assume you satisfy the assumptions that and and then find that . If the settings are not the exact same, you don’t know whether the non-equivalent estimates result from sampling variability, or a lack of external validity across different contexts. ↩︎To me, this is a question where the causal inference mindset and the policy evaluation mindset subtly diverge. If your goal is to recover the true causal effect
, then yeah, you need to assume none of your data are coming from a context where the treatment is inapplicable and irrelevant. Including such data would bias your estimate of for the domains where the treatment worked. Conversely if your goal is to estimate the utility of scaling-up some intervention, and you don’t think you are able to prospectively guess where the treatment will work, then you probably care about the “biased” effect, or the expected return of deploying the treatment in the places it’s likely to be deployed. ↩︎The included studies cover the LRA insurgency in Uganda (x6), the Russia-Georgia war in 2008, the Sierra Leone Civil War (x3), the Tajik Civil War, The Nepal Civil War, The Israel-Hezbollah War in 2006, World War II, The wars of Yugoslav succession, the Kosovo war, the Chechen Wars, the Kyrgyzstan Civil War, the broader Israel-Palestine conflict, and the civil war in Burundi (x2). The studies also cover what I would call non-commensurable populations—children who were/not conscripted into rebel armies, adults who were/not conscripted into rebel armies, street football players (?), heads of household, senior citizens, former enlisted soldiers in national militaries, nationally representative samples—which is relevant to point 3 above. ↩︎
Usually when we look at the assumptions for design based inference (e.g. parallel trends in Diff-in-Diffs) we are not testing whether the assumption is satisfied, but rather whether it is violated in some common and blatant way. ↩︎
But see: https://m.xkcd.com/552/ ↩︎
Scholars of Asia make this point all the time, and they are right. A study that “only” tells us something about the politics of Indonesia is capturing a phenomenon that affects about one in every thirty people on earth. For China or India, the figure is about one in every six people. ↩︎